Risk Assessment Analytics: Key to Financial Decision-Making
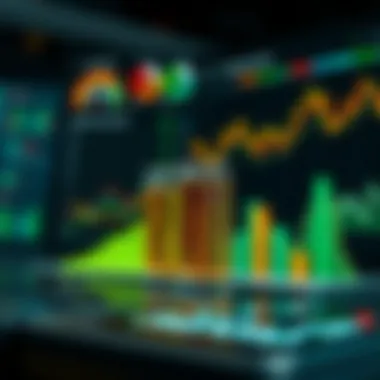
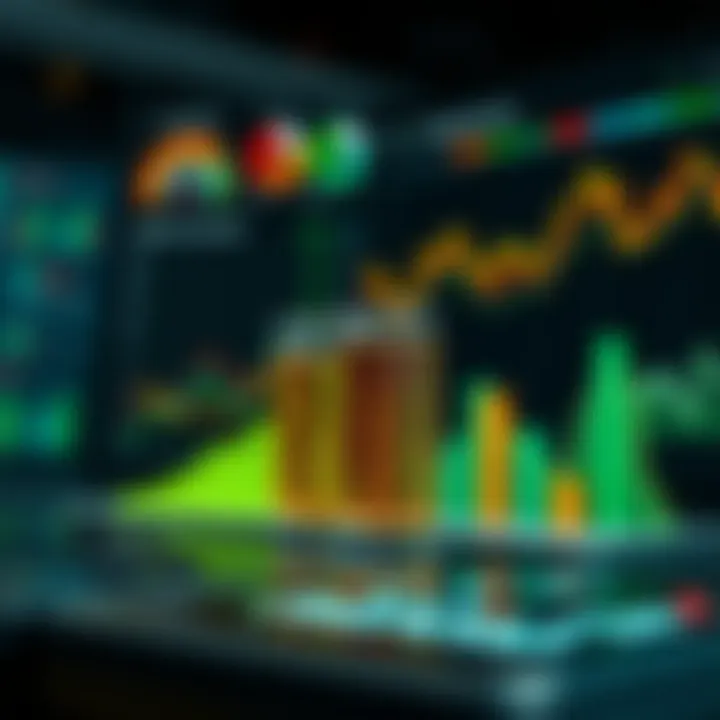
Intro
In today’s fast-paced financial landscape, the need for robust risk assessment analytics has never been more pronounced. As investors delve into complex markets, understanding the nuances of risk management is crucial for sound decision-making. This guide aims to unpack the intricacies of risk assessment analytics, shedding light on the tools and methodologies that empower both novice and experienced investors alike.
Navigating the financial waters involves more than a simple buy-and-sell strategy; it requires a keen understanding of potential pitfalls. Risk assessment analytics provides a structured approach to identifying, evaluating, and mitigating risks. By leveraging data and analytical techniques, financial professionals can make informed choices, ultimately bolstering investment performance.
As technology continues to reshape the financial sphere, it’s vital to stay abreast of these changes. Innovations such as artificial intelligence and machine learning offer powerful new avenues for analyzing risk, enabling investors to anticipate market fluctuations in ways that were once inconceivable. This article will explore how these advancements, alongside traditional methodologies, can cultivate a culture of informed financial decision-making.
Prologue to Risk Assessment Analytics
In the ever-evolving world of finance, understanding risk isn’t just a box to tick; it’s a necessity for survival and growth. Risk assessment analytics provides a structured approach to identify, evaluate, and manage potential pitfalls that can threaten financial stability. For both budding investors and seasoned professionals, diving into risk assessment analytics can be a game-changer.
Definition of Risk Assessment Analytics
At its core, risk assessment analytics involves collecting and analyzing data to evaluate risks that could impact financial outcomes. It’s not merely about identifying threats; it’s about quantifying them and applying a strategic lens through which decisions are made. In practical terms, this might involve using various statistical models and algorithms to assess things like market volatility, credit risk, and operational hazards. The real beauty of this process lies in its ability to turn complex data into actionable insights.
Importance in the Financial Landscape
The role of risk assessment analytics in the financial sphere cannot be overstated. Here are several reasons why it bears significance:
- Strategic Insights: It helps in crafting informed strategies that are tailored to the potential risks, leading to more accurate forecasting and resource allocation.
- Investment Protection: By understanding the landscape of risks, investors can safeguard their assets and better anticipate downturns, thereby minimizing losses.
- Regulatory Compliance: Financial institutions are often mandated to implement risk assessment practices. This not only promotes transparency but also fosters a sense of trust among stakeholders.
- Market Adaptability: Continuous analysis equips firms with the agility needed to respond to unforeseen challenges and capitalize on new opportunities. For instance, factors like emerging market trends or shifts in consumer behavior can be identified early on and integrated into risk models.
"In finance, knowing where the landmines are buried can save you from blowing up your portfolio."
This commitment to robust risk assessment ultimately contributes to a culture of responsible investment. By embedding risk assessment analytics into the fabric of financial decision-making, firms create a sustainable approach that balances opportunity and risk. The narrative we build around risk in finance shapes our strategies, our investments, and, ultimately, our success.
Framework of Risk Assessment Analytics
In the world of finance, having a solid framework for risk assessment analytics is akin to having a reliable compass when navigating a vast and unpredictable ocean. A well-structured approach allows investors and financial professionals to systematically analyze risks, make informed decisions, and, most importantly, protect their interests against uncertainties. This section will dig into the key components of risk assessment analytics, as well as the methodological approaches that define its practice.
Key Components
Understanding the framework starts by breaking it down into its essential components. Each element plays a pivotal role in building a robust risk assessment strategy. Let’s delve into these three key components in detail.
Risk Identification
Risk identification is the first step in the risk assessment journey. This process involves pinpointing potential hazards that could impact financial goals. The key characteristic of risk identification is its forward-looking nature; it aims to unearth possible threats before they materialize, allowing organizations to be proactive rather than reactive.
One unique feature of risk identification is its reliance on diverse data sources, which can include everything from historical data to industry trends. In essence, it thrives on an organization’s ability to gather information from various domains—this interdisciplinary approach can unveil risks that might go unnoticed if one solely relied on a singular data stream.
The advantages of this approach include enhanced agility in addressing potential threats and a more comprehensive understanding of the financial landscape. However, it does come with its own set of challenges. The efficacy of this process is highly contingent on the quality and breadth of the information collected. Thus, inadequate data can lead to a skewed perception of risk, potentially leading to catastrophic financial decisions.
Risk Evaluation
Once risks have been identified, the next step is risk evaluation. This point is crucial, as it determines the significance of the identified risks and their potential impact on the organization. Evaluating risks involves assessing the likelihood of their occurrence and the consequences they might yield, effectively establishing a risk profile.
The key characteristic here is quantification—measuring risks in numerical terms is fundamental to effective evaluation. The incorporation of statistical methods allows for a more empirical analysis of risk factors. A beneficial aspect of risk evaluation is its ability to prioritize risks based on their potential impact on financial stability. This prioritization becomes vital in resource allocation and strategic planning.
However, there's a downside as well. Quantitative models can sometimes oversimplify complex risks, potentially leading to a false sense of security. Moreover, they often depend on assumptions that, if flawed, can skew results, underlining the need for careful consideration in interpreting outcomes.
Risk Prioritization
After evaluating risks, the focus shifts to risk prioritization. This component is where decisions take shape, as it enables stakeholders to allocate resources effectively. The importance of prioritization lies in its ability to spotlight which risks deserve immediate attention and which ones can be monitored over time.
Key to this stage is the classification of risks into various tiers—high, medium, and low. This systematic categorization helps streamline the decision-making process. The unique feature here is that it encourages a strategic approach: addressing high-priority risks first can safeguard an organization’s financial health in the shortest time frame.
On the flip side, an overemphasis on prioritization may lead to neglecting lower-tier risks that can accumulate in their effect. This neglect can lead to missed opportunities for improvement or even risk mitigation. Thus, finding a balance between the urgency of response and the potential long-term risk landscape is crucial.
Methodological Approaches
Having established the framework's key components, we shall now explore the methodological approaches to risk assessment analytics. These approaches serve as the pathways through which the risks can be analyzed and managed, offering both qualitative and quantitative perspectives.
Qualitative Analysis
In qualitative analysis, professionals assess risks through subjective and descriptive methods. Unlike quantitative analysis, which relies heavily on numerics and statistical data, qualitative analysis leans into expert opinions, experiences, and judgment. This characteristic makes qualitative analysis beneficial for capturing nuances that numbers may overlook.
One unique feature of this methodology is its adaptability. It can be employed in various scenarios where quantitative data might be scarce or where newer risks emerge that aren’t fully understood. For instance, assessing emerging markets or novel financial instruments often requires insights that go beyond traditional metrics.
However, qualitative analysis does come with limitations. The subjectivity involved may introduce bias, leading to inconsistent assessments across different stakeholders. This variability underscores the importance of consolidating insights from multiple experts to glean a more rounded viewpoint.
Quantitative Analysis
Quantitative analysis serves as the counterbalance to qualitative methods, prioritizing numerical data to measure risks. This approach applies statistical methods to analyze trends, forecast potential outcomes, and ultimately, enables more precise risk modeling.
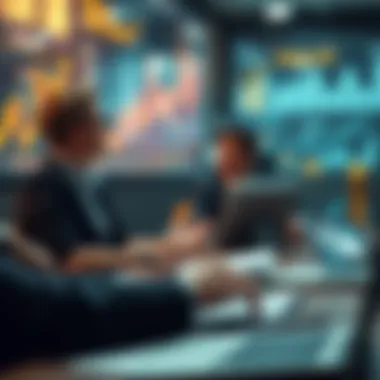
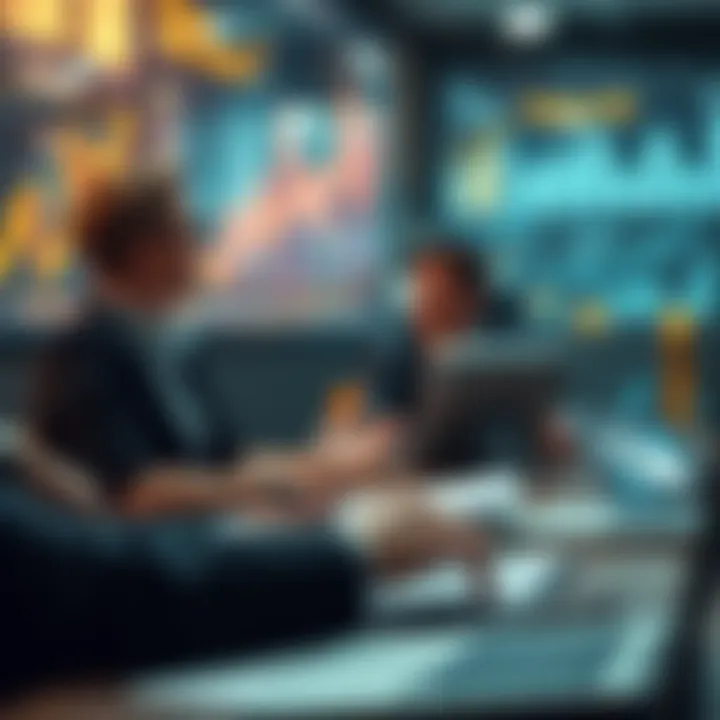
The method's key characteristic lies in its reproducibility—solid data gives consistent results that allow for reliable forecasts. This strength is especially advantageous when making data-driven decisions about investments and resource allocation.
Nonetheless, one must tread carefully. The risk models used can be complex, and miscalculations due to incorrect assumptions can lead to erroneous conclusions. Therefore, complementing quantitative analysis with qualitative insights often leads to more nuanced and informed decision-making, ensuring a well-rounded risk assessment.
Effective risk assessment is not merely about numbers; it's about understanding the story they tell.
By combining both the components and methodological approaches to risk assessment, investors and financial professionals acquire a powerful toolkit for navigating uncertainties in the financial landscape.
Tools and Techniques for Risk Assessment
In the intricate dance of finance, tools and techniques for risk assessment play a pivotal role. They help stakeholders sift through a mountain of data to understand potential pitfalls and opportunities alike. With a sound grasp of these tools, investors and financial professionals can make informed decisions that steer their investments wisely. This section delves into statistical tools and advanced technologies, offering insight into how they contribute to effective risk assessment analytics.
Statistical Tools
Analyzing the past can offer a treasure trove of insights when it comes to forecasting future trends in finance. Statistical tools are the compass guiding this analysis, with methods like regression analysis and Monte Carlo simulation leading the way.
Regression Analysis
Regression analysis is a statistical method that uncovers the relationships between variables. A fundamental aspect of this technique is its ability to predict outcomes based on previous data. Investors often employ regression analysis to understand how various factors—like interest rates or market trends—impact asset prices.
A key characteristic of regression analysis is its versatility. Whether dealing with linear regression, where relationships manifest in straight lines, or multiple regression that takes into account several influencing factors, this approach is robust. Its popularity lies in its accessibility and the actionable insights it provides. The unique feature of regression analysis is its ability to quantify relationships, offering investors a clear view of how one variable might drive changes in another.
However, it’s not without drawbacks. While regression analysis can highlight correlations, it can't confirm causation. A misinterpretation of the data might lead to incorrect assumptions. Still, the advantages it brings to the table—like clarity in forecasting and risk evaluation—make it a staple in risk assessment analytics.
Monte Carlo Simulation
Monte Carlo simulation is another statistical tool that offers profound insights, particularly in scenarios steeped in uncertainty. The method employs random sampling to model various outcomes, allowing analysts to evaluate the likelihood of different results. It’s akin to rolling the dice in a financial game, where various scenarios reveal potential risks and rewards.
The key characteristic of Monte Carlo simulation is its ability to incorporate variability in input assumptions, resulting in a wide range of possible outcomes. This dynamism is particularly beneficial in complex financial models where simplicity just won’t cut it. What sets Monte Carlo apart is this capability to simulate thousands, if not millions, of possible outcomes, painting a comprehensive picture of potential scenarios for investors.
On the flip side, the complexity involved in running these simulations can be a double-edged sword. The need for robust computational power and expertise can also be a barrier, especially for smaller firms. Nevertheless, when wielded correctly, Monte Carlo simulation can provide a solid foundation for informed decision-making in uncertain environments.
Advanced Analytical Technologies
As the landscape of finance evolves, so does the arsenal of tools available for risk assessment. Advanced analytical technologies like artificial intelligence and machine learning applications are revolutionizing how financial professionals assess risk.
Artificial Intelligence
Artificial intelligence (AI) has emerged as a significant game-changer in risk assessment. Its core capability lies in its ability to process vast data sets quickly and accurately, seeking patterns that would take a human analyst eons to uncover. This efficiency allows firms to react swiftly to market changes, enhancing their ability to manage risk effectively.
The key characteristic that makes AI particularly beneficial in this context is its capacity for predictive analytics. By analyzing historical data, AI systems can recognize trends and anticipate future events, informing risk strategies that are proactive rather than reactive. The unique feature of AI in risk assessment lies in its continuous learning—these systems improve over time, getting sharper with each data set processed.
Nevertheless, challenges exist. The reliance on data quality is paramount; less-than-stellar data can lead to faulty predictions. Plus, there’s a layer of complexity in understanding how AI models arrive at their conclusions, which can lead to issues in trust and transparency.
Machine Learning Applications
Machine learning, a subset of AI, is carving out its niche in risk assessment analytics. It employs algorithms that enable systems to learn from data and improve over time without being explicitly programmed. This adaptability is a boon for risk assessment, particularly regarding evolving market conditions.
The key characteristic of machine learning applications is their capacity for handling large data sets and discovering patterns that may elude traditional methods. Their ability to fine-tune analyses in real-time makes them an invaluable choice for financial professionals looking to gain a leg-up on competition. A distinguishing feature is their ability to create models that adapt and self-correct, ensuring that assessments are in tune with current trends.
However, machine learning isn't without its pitfalls. The complexities in model interpretation can be frustrating, and overfitting is a common concern— where a model works wonders on historical data but fails to predict future events effectively. Despite these challenges, the advantages machine learning brings in precision and efficiency make it an essential tool for modern risk assessment.
The integration of statistical tools and advanced technologies forms a comprehensive framework that significantly enhances risk assessment capabilities in finance.
By recognizing the merits and limitations of these tools, professionals can navigate the often-turbulent financial waters with greater confidence and strategic insight.
Data Sources for Effective Risk Assessment
In the realm of financial risk assessment, the backbone of robust analysis is essentially the data upon which it rests. To determine the precise nature and magnitude of risk, it's crucial to harness reliable data sources. Not every data point carries equal weight; hence, understanding the categorization of data sources sets the stage for more informed decisions. Relying on impeccable data not only mitigates risks but also enhances the overall decision-making process, leading to sound financial strategies.
The essence of effective risk assessment analytics lies within two major types of data: historical data and real-time data. Each type serves unique roles, bringing its own advantages and challenges into the fold of financial analytics. Let's delve deeper into each category to unearth their significance.
Historical Data
When speaking of risk assessment in finance, historical data acts as an architect of foresight. By evaluating past events, trends, and patterns, analysts can extract insights that illuminate possible future risks. Historical data can encompass a host of variables such as previous stock prices, economic indicators, or even outcomes of prior financial decisions.
The key characteristic of historical data is its track record. Investors often rely on this data to analyze how similar circumstances led to various outcomes, thus allowing them to draw parallels and make predictions. It provides a foundation upon which future forecasts are built.
However, caution must be exercised. While historical data carries undeniable value, it does not guarantee that future scenarios will mirror those from the past. These derived trends can be misleading if not cautiously interpreted. Thus, while historical analysis informs risk models, it should be supplemented with other modern approaches to provide more holistic insights.
Real-time Data
The pulse of today's financial markets is real-time data. This dynamic data captures current trends, market fluctuations, and immediate consumer behaviors. Engaging with this data allows investors and financial professionals to react promptly to emerging risks.
Market Trends
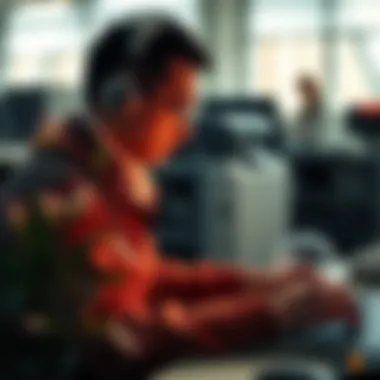
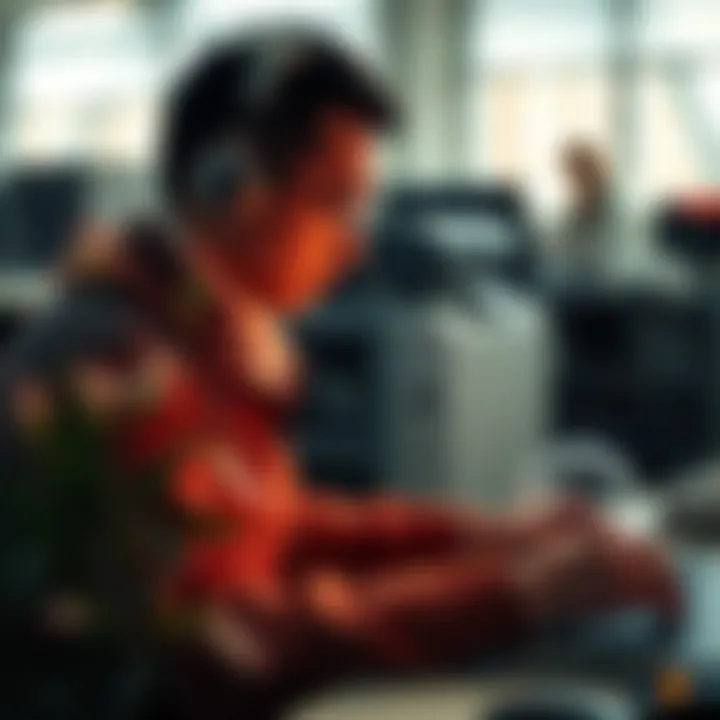
Market trends play an essential role in understanding the current financial landscape. By mapping out patterns in trade volumes, price movements, or sector performance, investors can identify shifts that signal potential risks or opportunities. This aspect of market trends is particularly beneficial as it captures volatility in the market, often enabling investors to pivot strategies ahead of larger market movements.
A unique feature of market trends is their immediacy; they reflect current sentiment and behavior, making them indispensable for short-term decision-making. By integrating market trends into risk assessment analytics, investors can vastly improve their responsiveness to potential risks. However, it’s important to balance this focus on immediacy with contextual understanding. Market noises can heavily distort signals, resulting in impulsive decisions if not properly filtered.
Consumer Behavior
Consumer behavior is another vital aspect of real-time data that profoundly shapes risk assessment. Analyzing how consumers respond to market shifts, pricing changes, or economic conditions can provide insights into future risks. The key characteristic of consumer behavior data is its reflective nature—it mirrors the collective sentiment of the market.
This data source is advantageous as it allows companies to anticipate challenges and adjust accordingly. For instance, if a sudden dip in consumer confidence is reported, investors can take proactive measures to stave off potential losses. However, the caveat lies in the rapidly shifting nature of consumer sentiments, which can sometimes lead to volatile predictions.
In summary, harnessing a blend of historical and real-time data is indispensable for effective risk assessment analytics. Not only does it provide a comprehensive view of potential risks, but it also enables investors to make decisions backed by empirical evidence.
"Data is the new oil, but like oil, it must be refined to be useful."
Effective utilization of these data sources is instrumental in crafting a roadmap for navigating financial uncertainties.
Implications of Risk Assessment Analytics
Risk assessment analytics plays a pivotal role in shaping the financial landscape by providing essential insights into the nature, likelihood, and impact of potential risks. Understanding these implications can significantly enhance decision-making processes and support strategic risk management. In today’s market, where uncertainty breeds volatility, the effective application of these analytics becomes crucial for both investors and institutions alike.
Enhancing Decision-Making Process
Risk assessment analytics serves as a guiding light in decision-making, enabling investors to make informed choices. By analyzing historical data and current trends, financial professionals can identify patterns that may foreshadow future market behaviors. This analytical approach helps in choosing investment opportunities wisely.
One key aspect is the ability to quantify risks associated with various investment strategies. This quantification allows stakeholders to weigh the potential returns against the risks, providing clarity that can foster confidence in decision-making. With risk assessments clearly laid out, investors are less likely to be swayed by market noise and can instead focus on long-term strategies. Without a structured analysis, investors might chase after trends that could lead them down a rabbit hole of losses.
Moreover, the data-driven nature of these analytics promotes a culture of accountability. Having concrete data to back decisions helps mitigate the risks of subjective judgment, ensuring that choices align with strategic objectives instead of mere gut feelings.
Strategic Risk Management
Investors can adopt a proactive approach to risk through strategic risk management, backed by robust analytics. By identifying and evaluating the risks at play, these tools allow financial professionals to formulate strategies tailored to minimize potential setbacks.
Mitigating Financial Losses
Mitigating financial losses is where risk assessment analytics truly shines. It allows organizations to establish protocols aimed at reducing the financial fallout from adverse risks. By employing advanced tools like Monte Carlo simulations, analysts can foresee potential scenarios and their financial implications, which helps in crafting sound strategies to buffer against losses.
This proactive approach not only safeguards capital but also helps in maintaining investor trust. Financial institutions that successfully mitigate losses present themselves as stronger players in the field, boosting their reputation in a competitive market. It's worth noting, however, that the effectiveness of loss mitigation strategies really hinges on the quality of the data used. Poor-quality data can lead to weak or ineffective strategies, further complicating the risk landscape.
Preparing for Market Volatility
In an era of unpredictable economic landscapes, preparing for market volatility has become vital. The capacity to anticipate potential fluctuations provides financial entities with a significant edge. Risk assessment analytics facilitates this anticipation by using models that identify high-risk environments and displaying possible outcomes should market conditions change swiftly.
One unique feature of preparing for volatility is that it allows firms to establish contingency plans. A well-informed plan that takes volatility into account can lead to faster recovery from market shocks. The advantage here lies in enabling financial professionals to not just react, but to also strategize ahead of time, thereby safeguarding their interests against sharp market downturns.
Ultimately, while strategies for managing volatility hold benefits, they also come with challenges. Market conditions can shift unpredictably, and relying solely on past data and trends might not always yield positive results in future circumstances. It’s important that financial professionals remain agile and adapt their strategies to respect the ever-changing market variables.
Challenges in Risk Assessment Analytics
Risk assessment analytics serves as a compass for navigating the financial seas, but it's not all smooth sailing. The field faces its fair share of challenges that can muddy the waters. Understanding these hurdles is essential, not just for financial professionals but also for anyone engaging with the intricacies of investment. By shining a light on these trouble spots, we can better appreciate the importance of solid analytics in making informed decisions.
Data Quality Issues
Data is the backbone of risk assessment analytics, and without high-quality data, the entire framework can collapse faster than a house of cards. It's like trying to build a beautiful sandcastle with sandy soil; no matter how great your design is, if the sand isn’t right, it’ll crumble.
- Sources & Integrity: Gathering information from multiple sources, such as market reports, social media analytics, and historical records, can introduce discrepancies. Each source holds its unique biases or errors, which can distort the overall picture.
- Timeliness: Market conditions change like the wind, which means data must be fresh. Old data can yield outdated insights, affecting risk evaluations. When dealing with financial markets, a lag can often mean a missed opportunity or significant loss.
- Transformation Errors: The step where data is cleaned and transformed is crucial. A small mistake here can lead to massive miscalculations later on, skewing the risk assessment entirely.
It’s clear that maintaining high data quality is non-negotiable. Investors should establish stringent data governance practices to ensure accuracy. Collaborating with reputable data providers can also help establish credibility.
Complexity in Risk Models
Risk models are the tools through which risk analytics operates, yet they can often be obtuse, like deciphering an ancient script. The complexity arises from the numerous variables involved, and managing them requires not only expertise but also a clear understanding of their interdependencies.
- Model Specification: Designing a model that captures all relevant factors while remaining manageable is no easy task. Striking the right balance between simplicity and comprehensiveness often feels like walking a tightrope.
- Algorithm Flaws: Even the best algorithms can have blind spots. If the algorithm lacks the appropriate variables or assumptions, it might produce results that are far from reality. This situation may give investors a false sense of security or an exaggerated perception of risk.
- Interpreting Outputs: Once the analysis is complete, interpreting what the numbers imply can be challenging. A complex model might yield all kinds of metrics, but without the know-how, an investor may misread those indicators.
Effectively addressing these challenges involves continuous learning and adaptation. Financial professionals must engage with ongoing education and seek out expert opinions to mitigate risks stemming from these complex models. Collaborating with analytics specialists can also enhance understanding and lead to more reliable assessments.
"In the world of finance, understanding risk is not just about metrics; it’s about connecting the dots in a sometimes chaotic landscape."
By confronting these challenges head-on and implementing effective strategies, investors and financial analysts can pave the way for more reliable and insightful risk assessment analytics, ultimately fostering a more resilient financial environment.
Case Studies on Risk Assessment Analytics
In the realm of finance, practical applications often speak louder than theory. Case studies on risk assessment analytics showcase real-world scenarios, providing valuable insight into how institutions tackle risk effectively. They reveal not just successes, but also the pitfalls along the way, offering a blueprint for others to follow, or perhaps avoid. Such case studies allow financial professionals to anchor their theoretical knowledge in tangible outcomes, bridging the gap between strategy and execution.
Successful Implementations
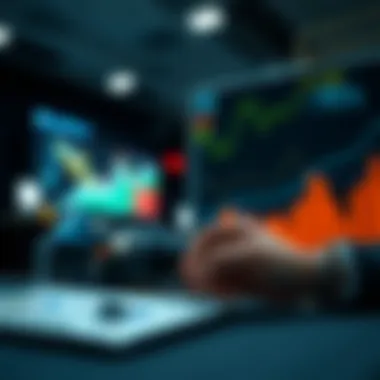
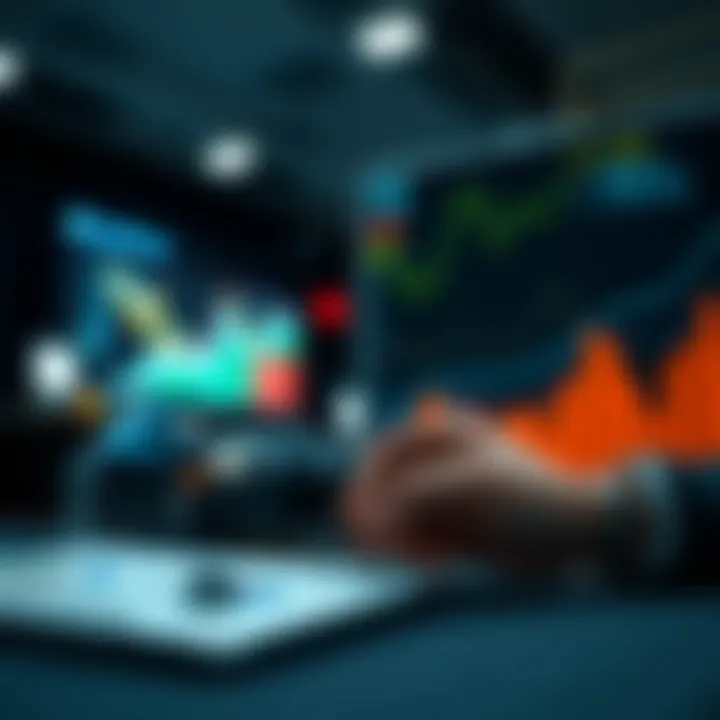
To appreciate the successful implementations of risk assessment analytics, one needs to look at specific instances that demonstrate the vital role these practices play in finance. A notable example is Citibank, which integrated advanced risk analytics into their credit assessment process. By leveraging predictive modeling, they improved their ability to assess credit risk substantially. This gave them an advantage in decision-making, helping prevent potential losses that could arise from bad debts.
Another excellent case comes from Wells Fargo, which utilized data integration tools to amalgamate information from various sources. By employing real-time data analytics, they were able to identify and prioritize risks associated with different investment portfolios more effectively. This enabled them to mitigate potential losses and enhance their overall risk management strategy.
The success of these companies emphasizes the importance of adopting a rigorous approach to data analysis and risk assessment. With well-formulated strategies, financial institutions can navigate through complexities and uncertainties far more adeptly than others lacking such frameworks.
Lessons Learned
While these success stories inspire, they also provide crucial takeaways for those looking to implement similar systems. Here are some lessons learned from these case studies:
- Data is Key: Quality of data impacts the efficacy of risk assessment analytics significantly. Both Citibank and Wells Fargo highlighted the importance of accurate and up-to-date data as foundational for any analysis.
- Continuous Monitoring is Essential: The financial world is in constant flux. As illustrated by Wells Fargo, ongoing evaluation and adaptation of risk models ensure that institutions remain responsive to market changes and internal dynamics.
- Integration of Diverse Approaches: A lone strategy can lead to blind spots. Successful case studies show the significance of merging qualitative insights with quantitative data. This holistic view often leads to a better understanding of risk profiles.
- Training and Culture: Both success cases underscore the importance of fostering a culture of risk awareness. Employees need not just the right tools but an understanding of how to use them, cultivating a more informed workforce.
As these lessons demonstrate, while strategies and tools for risk assessment analytics might evolve, the foundational approaches remain remarkably consistent. Financial professionals must stay adaptable, learning from real-world applications, to navigate the ever-changing landscape of risks in finance.
"Success leaves clues." – This is especially true in the realm of risk assessment analytics. As you analyze these case studies, remember that the pitfalls are as instructive as the triumphs.
Future Trends in Risk Assessment Analytics
The financial sector is undergoing significant changes. As the complexities of global markets grow, so does the need for robust risk assessment analytics. Understanding the future trends in this field is crucial for investors and financial professionals. This section will highlight the trends that can shape decision-making processes and strategies in the coming years.
Integration of Blockchain Technology
Blockchain technology is not just a buzzword; it’s becoming a cornerstone in risk assessment analytics. By providing a decentralized and transparent way to track transactions, blockchain holds promise to transform how risk is evaluated in finance. It ensures data integrity, which is paramount for effective risk analysis. For instance, in trading platforms, transactions recorded on a blockchain can substantially reduce discrepancies. This makes the detection of potential fraud far easier.
Furthermore, the immutability of blockchain records helps maintain a clear audit trail. Financial institutions can thus perform risk assessments on a well-documented history of transactions. Investors benefit as they gain greater trust in the data driving risk assessment models.
- Key benefits include:
- Enhanced transparency in transactions
- Immediate accessibility of data for audits
- Reduced costs associated with third-party verifications
In sum, the integration of blockchain technology can revolutionize risk management by fostering trust and accountability.
Evolving Regulatory Landscape
Financial markets are heavily regulated, and understanding the evolving regulatory landscape is crucial for risk assessment analytics. Regulatory bodies continuously update requirements to ensure market integrity and protect investors. For instance, laws aimed at combating money laundering and fraud put pressure on companies to enhance their risk assessment frameworks.
Moreover, new regulations about data privacy, like the General Data Protection Regulation (GDPR) in Europe, challenge financial institutions to navigate compliance and risk simultaneously. This necessity compels companies to invest in advanced analytics to not only adhere to regulations but also to identify and mitigate any associated risks.
"As compliance requirements evolve, firms must adapt their risk assessment strategies to avoid penalties and maintain credibility."
Investors and professionals need to stay updated on upcoming changes, as these can significantly alter risk dynamics. Considerations include:
- Active participation in industry forums on regulatory impacts
- Utilizing adaptive risk assessment frameworks that allow quick responses to regulatory updates
- Engaging with regulatory technology solutions to streamline compliance processes
As regulations develop, it is clear that staying ahead of these trends will influence overall market stability and informed investing.
Best Practices for Implementing Risk Assessment Analytics
Implementing risk assessment analytics effectively can significantly influence an organization's success in navigating financial uncertainties. By adhering to best practices, financial professionals can cultivate a structured approach that enhances decision-making, supports strategic risk management, and leads to optimal outcomes. The following points spotlight the significance of these practices:
- Clarity: Establishing a clear framework streamlines discussions and ensures everyone is on the same page.
- Goal Orientation: A focus on objectives brings discipline to the analysis process, helping prevent common pitfalls and missteps.
- Adaptability: The financial landscape is constantly shifting, and so must the methods of risk assessment.
In the following sections, we will drill down into two key best practices: how to establish clear objectives and the importance of continuous improvement and monitoring.
Establishing Clear Objectives
A well-defined objective acts as the compass guiding the risk assessment effort. Financial organizations often find themselves exploring vast amounts of data; having specific goals in place can ensure they don't just go fishing in the dark.
When setting these objectives, consider the following elements:
- Specificity: Clearly articulate what you aim to achieve from the risk analytics process. Whether it's identifying credit risk or assessing market volatility, being precise pays dividends.
- Measurable Outcomes: Define metrics that will help gauge success, pivoting as necessary based on real-world results. Tools like KPIs can be quite handy in this respect.
- Relevance: Ensure that objectives are aligned with the broader organizational strategy and goals. This keeps the analysis pertinent and actionable.
- Time-bound: Establish deadlines. Without a time frame, analysis can drag on endlessly, diluting the urgency and relevance of findings.
These considerations help carve a path for effective risk management. For example, a banking institution may aim to reduce credit default risk by 15% within the next quarter. Specificity in terms makes it easier to isolate factors contributing to risk and devise solutions that can be tracked over time.
Continuous Improvement and Monitoring
Risk assessment is not a one-time affair; it requires ongoing vigilance and a commitment to improvement. Established practices should evolve alongside the market, technology, and regulatory changes. Continuous monitoring is the song that keeps playing, and here’s why it matters:
- Proactive Adjustments: By consistently reviewing metrics and outcomes, companies can quickly identify areas needing tweaking. Let’s say you notice an uptick in fraud claims; this immediate feedback enables you to develop countermeasures swiftly.
- Learning from Mistakes: An organization's response to errors can define its success. Regular evaluations can uncover flaws in risk strategies, providing essential insights for improvement.
- Technological Trends: Staying abreast of advancements in analytics tools allows firms to leverage emerging capabilities, enhancing their risk assessment processes.
To support this, here's a practical approach to ensure effective monitoring:
- Scheduled Reviews: Periodic assessments should be non-negotiable. Regularly analyze results against predefined objectives.
- Feedback Loops: Incorporate insights from various stakeholders to enrich the assessment process. This helps in not just identifying weaknesses but also celebrating achievements along the way.
- Documentation: Keep records of findings and changes made. This not only aids in compliance but also serves as a learning tool for future iterations.
Implementing these best practices sets the stage for nimble and informed financial decisions, essential in a landscape where change is the only constant. By establishing clear objectives and committing to continuous improvement, organizations can streamline their risk assessment analytics and keep ahead of the curve.
"Effective risk management is less about avoiding risks and more about understanding and managing them."
Through diligent application of these practices, financial institutions can build resilience against uncertainties, ensuring they thrive no matter what the future holds.
Learn more about risk management strategies through Investopedia and see how leading organizations implement robust frameworks for effective decision-making.